Mathematical Statistics for Data Science
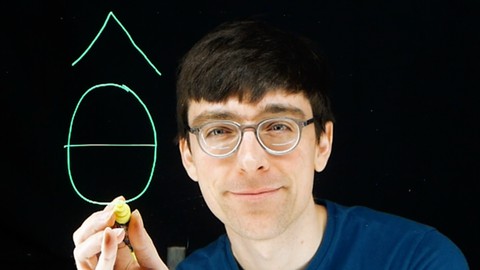

Price: 19.99$
This course teaches the foundations of mathematical statistics, focusing on methods of estimation such as the method of moments and maximum likelihood estimators (MLEs), evaluating estimators by their bias, variance, and efficiency, and explore asymptotic statistics, including the central limit theorem and confidence intervals. Course Highlights:57 engaging video lectures, featuring innovative lightboard technology for an interactive learning experience In-depth lecture notes accompanying each lesson, highlighting key vocabulary, examples, and explanations from the video sessions End-of-chapter practice problems to solidify your understanding and refine your skills from the course Key Topics Covered: Fundamental probability distributions: Bernoulli, uniform, and normal distributions Expected value and its connection to sample mean Method of moments for developing estimators Expected value of estimators and unbiased estimators Variance of random variables and estimators Fisher information and the Cramer-Rao Lower Bound Central limit theorem Confidence intervals Who This Course Is For: Students with prior introductory statistics experience, looking to delve deeper into mathematical foundations Data science professionals seeking to refresh or enhance their statistics knowledge for job interviews Anyone interested in developing a statistical mindset and strengthening their analytical skills Pre-requisites: This course requires a solid understanding of high school algebra and equation manipulation with variables. Some chapters utilize introductory calculus concepts, such as differentiation and integration. However, even without prior calculus knowledge, those with strong math skills can follow along and only miss a few minor mathematical details.